Deepfake Identifier
Nowadays, the identification between an original and a deepfake image/video is a bit hard to make. But the computer scientists from the University of Buffalo have come up with an automatic identifier that differentiates between deepfake photos and original ones by analyzing light reflection in eyes.
In a paper accepted at the IEEE International Conference on Acoustics, Speech and Signal Processing, the tool proved to be 94% effective with portrait-like photos in experiments that are to be held in June in Toronto, Canada.
How Does It Work?
Siwei Lyu, Ph.D., SUNY Empire Innovation Professor in the Department of Computer Science and Engineering said that the cornea is almost like a perfect semi-sphere and is very reflective. The cornea surface will capture the image of anything that is coming to the eye with a light-emitting from those sources.
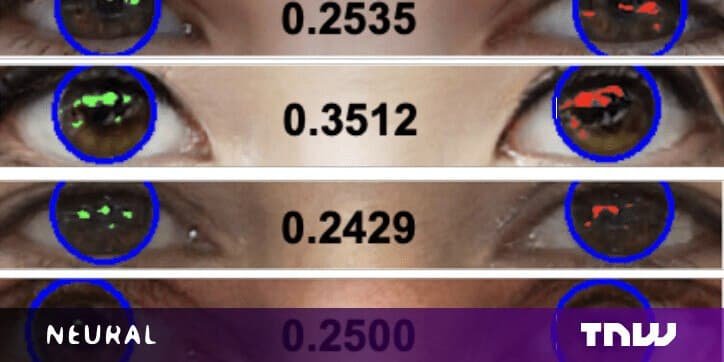
And obviously, an eye belonging to the same person will be watching the same object hence developing similar reflective patterns. Lyu said that it is not the very first thing observed by anyone in daily life.
The paperis available on the open-access repository arXiv with the title of “Exposing GAN-Generated Faces Using Inconsistent Corneal Specular Highlights.”
The partners in the research are Shu Hu and Yuezun Li with the former being a third-year student of computer science in Ph.D. and the latter being a former senior research scientist at UB.
How Easy to Identify reflection?
So it may be possible that reflections are formed on the eye but are they easily identifiable through clicked original or deepfake photos? The answer is yes as the reflections on the eyes would generally appear to be the same shape and color. So here is where generative adversary network (GAN) images fail in the test due to many photos combined to generate the fake image. Tiny deviations are spotted with the help of Lyu’s tool exploits in reflected light in the eyes of deepfake images.
Development of the system was a tricky part but the more tricky part was testing the system as real images from Flickr Faces-HQ were obtained along with fake images from www.thispersondoesnotexist.com, a repository of AI-generated faces that look lifelike but hence fake. All the images being tested were in portrait mode i.e., looking straight to the camera of 1024*1024 pixels.
Mapping out each face marks the start of the tool and then moves to examine eyes so that it can study the eyeball’s reflection individually. Potential differences in shape, light intensity, and other features of the reflected light are compared for the image.
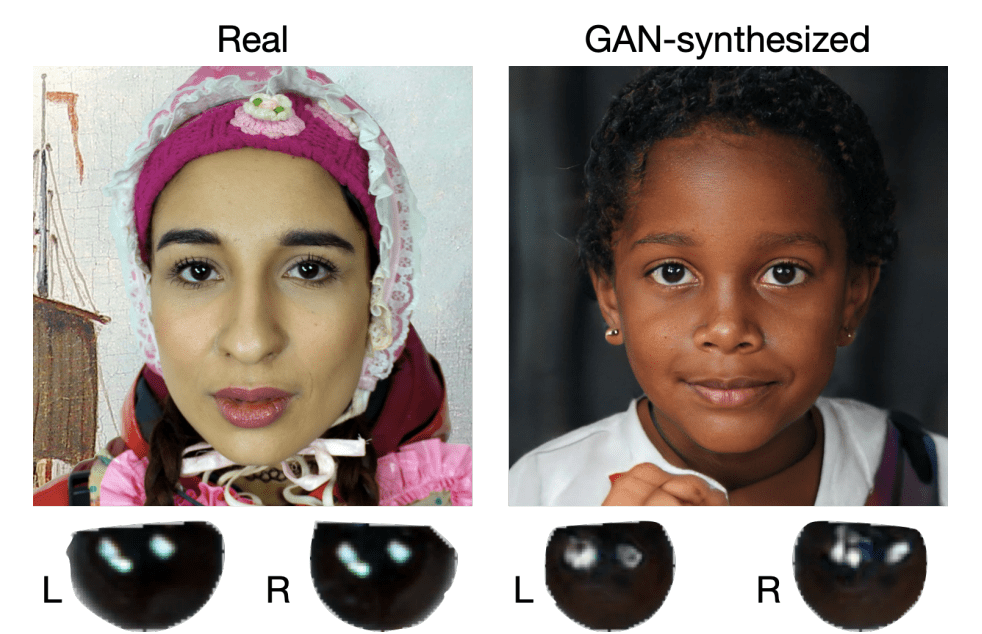
Limitations
The first one being that a reflected light source is needed. Secondly, the difference in the light reflection in eyeballs can be adjusted during image editing. Additionally, individual pixels reflected in the eyes are being looked at rather than the shape of the eye or the nature that is being reflected or shapes within the eyes.
And the last limitation is that if the object’s eye is missing or not visible then the technology fails as only the reflections within both eyes are compared.
Lyu spotted and proved that deepfake videos tend to have inconsistent or nonexistent blink rates for the video subjects, all due to his experience in machine learning and computer vision projects for over 20 years.
He is also for responsible developing the “Deepfake-o-meter” in association with Facebook in 2020 which is an online resource to help the average person test to see if the video they’ve watched is, in fact, a deepfake.
Unjustifiable Use
Lyu takes responsibility to clear winds around the deepfake as they are misleading and provide misleading information that could result in serious violence breakouts and he wants to control the use of deepfake as they have been used for pornographic content more.