According to a new study published in the Journal Heart, a modern machine-learning method of artificial intelligence (AI) can accurately predict the probability of cardiac arrest — heart attacks — using timing and weather info.
It’s important to remember, though, that the latest machine learning app can’t determine when people will die of cardiac arrest. It simply indicates that the probability of a heart attack is likely to increase.
Machine learning is the study of computational algorithms that are based on the premise that systems can learn and develop themselves from data by finding patterns and adapting with little or no human interaction. The study discovered that the risk of cardiac arrest outside of the hospital was greatest on Sundays, Mondays, after sudden temperature drops between days, and on public holidays.
According to the researchers, the latest results could act as an early warning system, lowering the risk of deadly episodes and increasing survival odds, as well as enhancing emergency medical providers’ capacity to brace for serious circumstances. This is notable because out-of-hospital cardiac arrests are frequent around the world and are associated with a poor survival rate.
Climate conditions worsen the risk even more. Although meteorological data is complicated, Japanese researchers believe that machine learning will inevitably uncover connections that traditional or one-dimensional statistical approaches will miss.
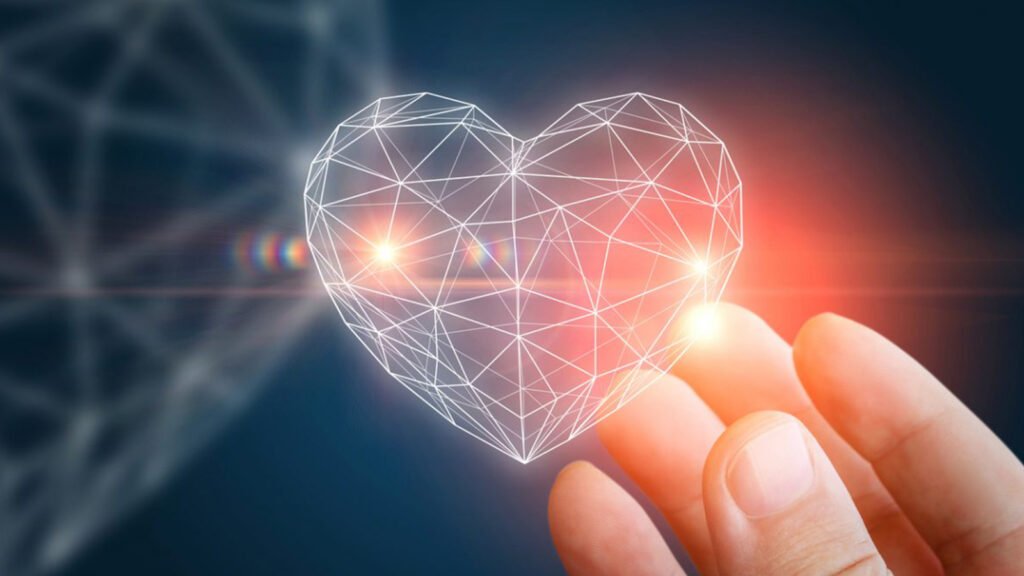
Further to their investigation, the researchers looked into how well machine learning could predict regular out-of-hospital cardiac arrests based on timing, such as the year, season, day of the week, hour of the day, or public holidays, as well as daily weather, such as relative humidity, snowfall, rainfall, temperature, wind speed, cloud cover, and air pressure readings.
Between 2005 and 2013, 1,299,784 cases occurred, and machine learning was used for 525,374 of them as a training dataset, using timing info, temperature, or both. These findings were then compared to 135,678 cases that occurred between 2014 and 2015 in order to evaluate the model’s ability to accurately predict the number of daily cardiac arrests in other years.
The researchers used a separate dataset taken from out-of-hospital cardiac arrests in Kobe city between January 2016 and December 2018 to perform a “heatmap survey” to expose the precision of this method at the local level. On both the research and instructional datasets, combining timing and weather data resulted in high accuracy of cardiac arrest predictions for out-of-hospital cases.
The “hotspots” of cardiac arrests on Sundays, Mondays, low temperatures, sudden rises in temperatures, winter, and public holidays were discovered by merging weather and timing details. However, the researchers claim to lack evidence about people with pre-existing medical problems and do not have detailed knowledge regarding the location of heart attacks outside of Kobe city. Both unusual circumstances may have influenced the outcome.
“Our predictive model for daily incidence of [out of hospital cardiac arrest] is widely generalizable for the general population in developed countries because this study had a large sample size and used comprehensive meteorological data,” said the researchers in an embargoed release shared with Interesting Engineering.
“The methods developed in this study serve as an example of a new model for predictive analytics that could be applied to other clinical outcomes of interest related to life-threatening acute cardiovascular disease,” added the researchers. “This predictive model may be useful for preventing [out of hospital cardiac arrest] and improving the prognosis of patients […] via a warning system for citizens and [emergency medical services] on high-risk days in the future.”
2 Comments
Pingback: PornHub remastered the oldest Erotic videos using AI - Craffic
Pingback: Pentagon developing AI Network which can predict the future events - Craffic